Pricing Models for AI Agents
by Curtis Duggan, Founder
Consider, if you will, the peculiar temporal moment in which we find ourselves: artificial minds—if we can call them that, and there's a whole separate discourse about whether we should—are beginning to do real work in the world. Not the parlor-trick kind of work that characterized early AI demos (though we still see plenty of those), but genuine, valuable, human-like labor that creates actual economic value in the world. Which brings us to the thorny question that nobody seems to want to address head-on: how do we price these digital laborers?
1. The Labor Model: An Exercise in Digital Economics
There's something almost comically straightforward about pricing AI Agents like human labor, as if we could simply transpose the entire apparatus of human economic value—hourly rates, overtime, the whole nine yards—onto these silicon workers. And yet (and here's where it gets interesting) this approach has a certain elegant logic to it. When you're replacing or augmenting human work, why not price it like human work? Just, you know, cheaper.
The beauty of this model lies in its familiarity. Everyone—from the CEO down to the newest intern—understands the basic calculus: time equals money. The complexity comes in when you start to really think about what "time" means for an AI. Does an AI Agent that can process a thousand documents in parallel take more or less time than one processing them sequentially? And who, exactly, is saving time here? These are the kind of metaphysical questions that keep pricing strategists up at night, their spreadsheets glowing in the dark like digital rosetta stones.
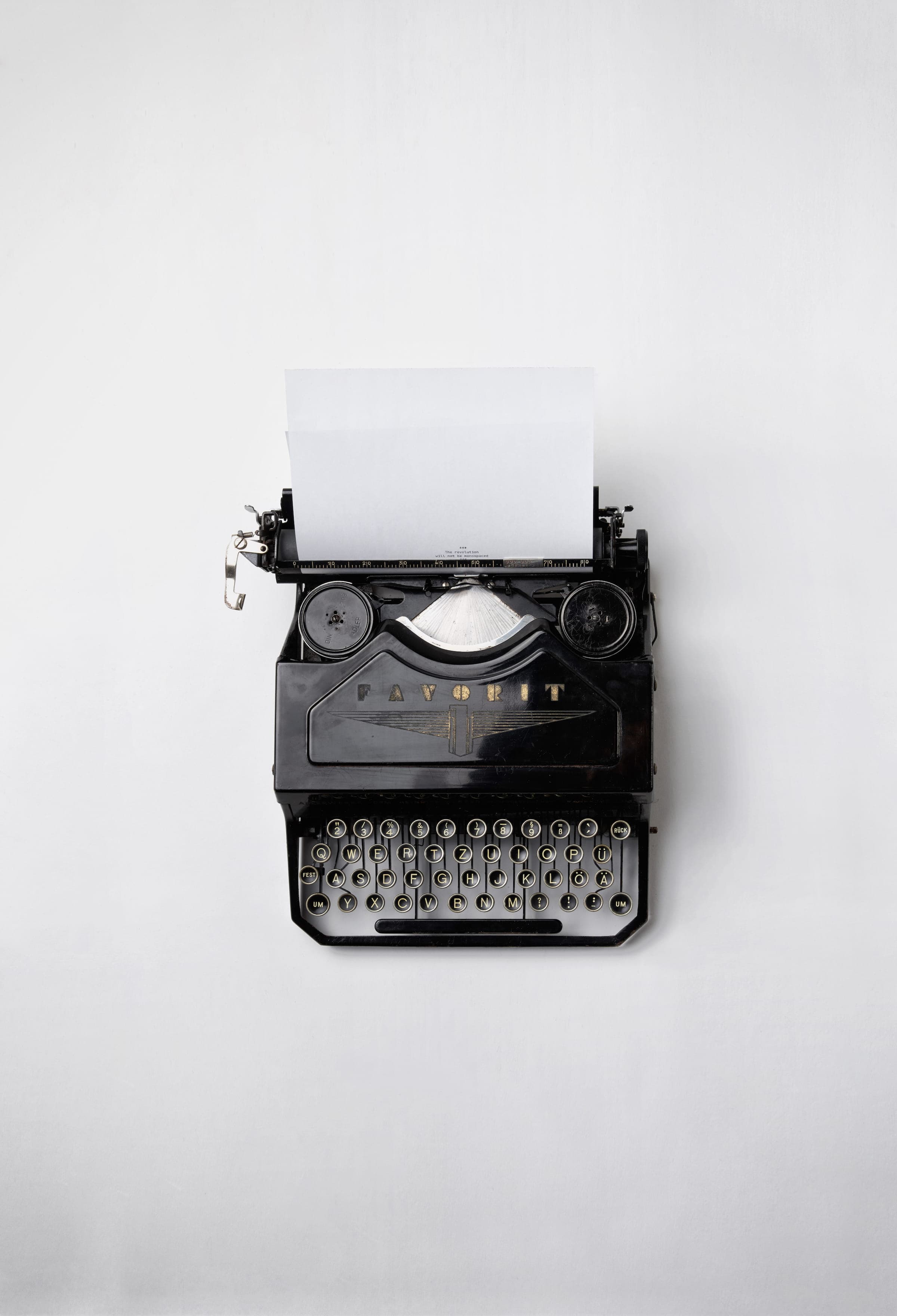
2. Outcome-Based Pricing: The Promise of Pure Value
Then there's the seductive simplicity of outcome-based pricing, which seems at first glance to cut through all the philosophical knots of the labor model like Alexander's sword. You want X? We'll charge you Y. Clean, simple, done. Except—and isn't there always an except?—it turns out that defining outcomes in the messy real world is about as straightforward as nailing jelly to a wall.
Consider the case of an AI Agent tasked with improving customer service responses. What's the outcome we're measuring? Response speed? Customer satisfaction? Problem resolution rates? And what happens when these metrics start to conflict with each other, as they inevitably do? You might find yourself in the peculiar position of having created an AI that's technically meeting all its metrics while somehow missing the whole point of customer service entirely.
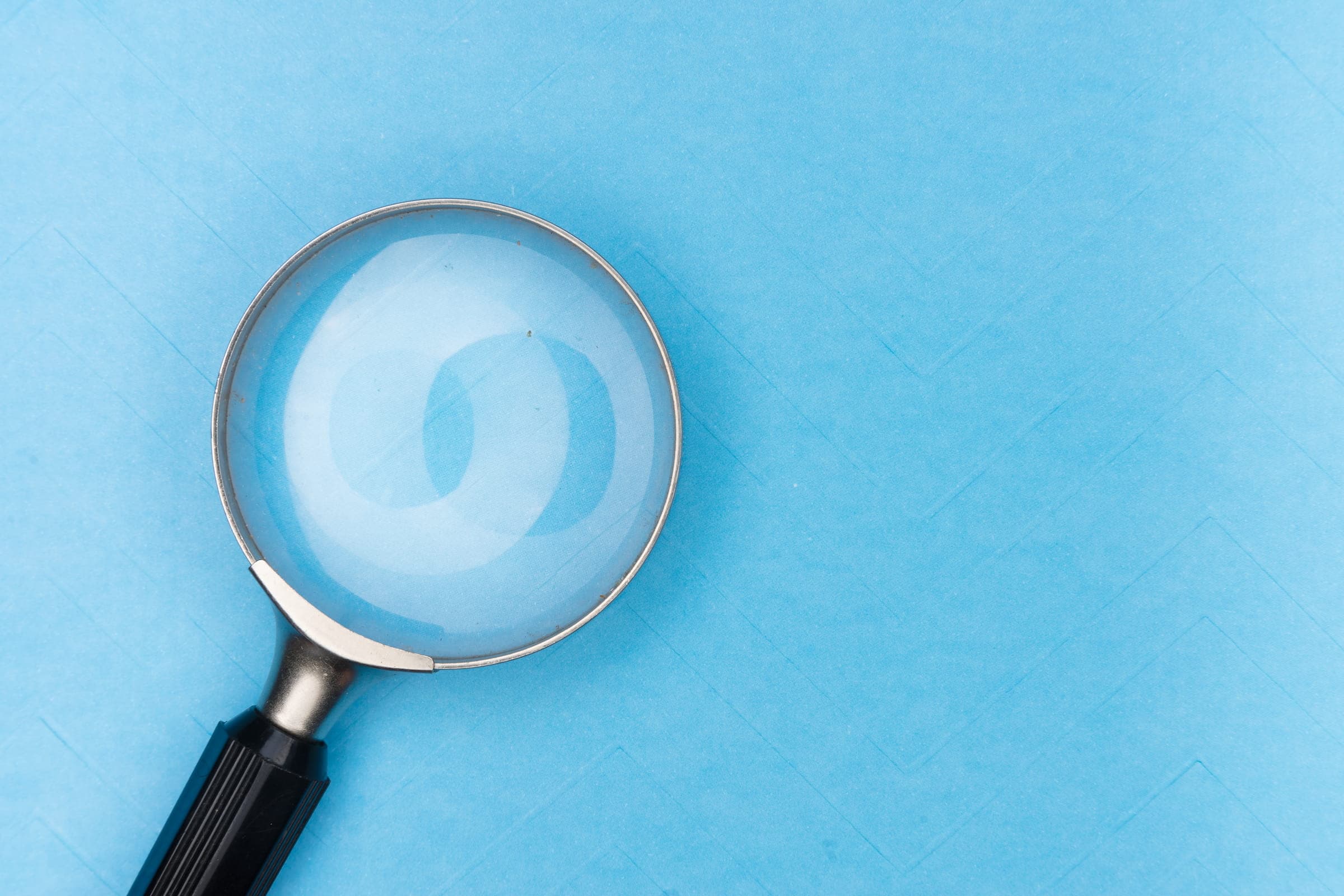
3. The Cost-Plus Model: A Tale of Transparency and Trust
Perhaps the most radical approach—and I use "radical" here in its original sense of "going to the root"—is to simply price these agents based on their actual cost plus a modest markup. This is the kind of pricing model that appeals to engineers and other technical types who appreciate its crystalline logical structure. It's also, not coincidentally, the kind of model that makes business strategists break out in a cold sweat.
The appeal here is obvious: complete transparency. You know exactly what you're paying for, down to the individual token. But there's something almost naively utopian about this approach, as if by making the economics transparent we could somehow transcend them. It's worth noting that no other industry prices its products based purely on cost—not even utilities, those paragons of regulated pricing, go quite this far.
4. The SaaS Seat Model: An Old Solution to a New Problem
And finally, we arrive at what might be called the conservative option: just treat AI Agents like any other software feature and roll them into a standard SaaS pricing model. There's something almost comforting about this approach, like putting a revolutionary new technology into a familiar old suit. But it's worth asking whether this comfort comes at the cost of missing something fundamental about what makes AI Agents different from traditional software.
The seat model works beautifully in certain contexts—particularly in organizations where you have many users each making moderate use of the AI. But it starts to break down in high-usage scenarios, where a single "seat" might be consuming massive amounts of computational resources. It's like trying to price electricity based on how many light switches you have, rather than how much power you actually use.
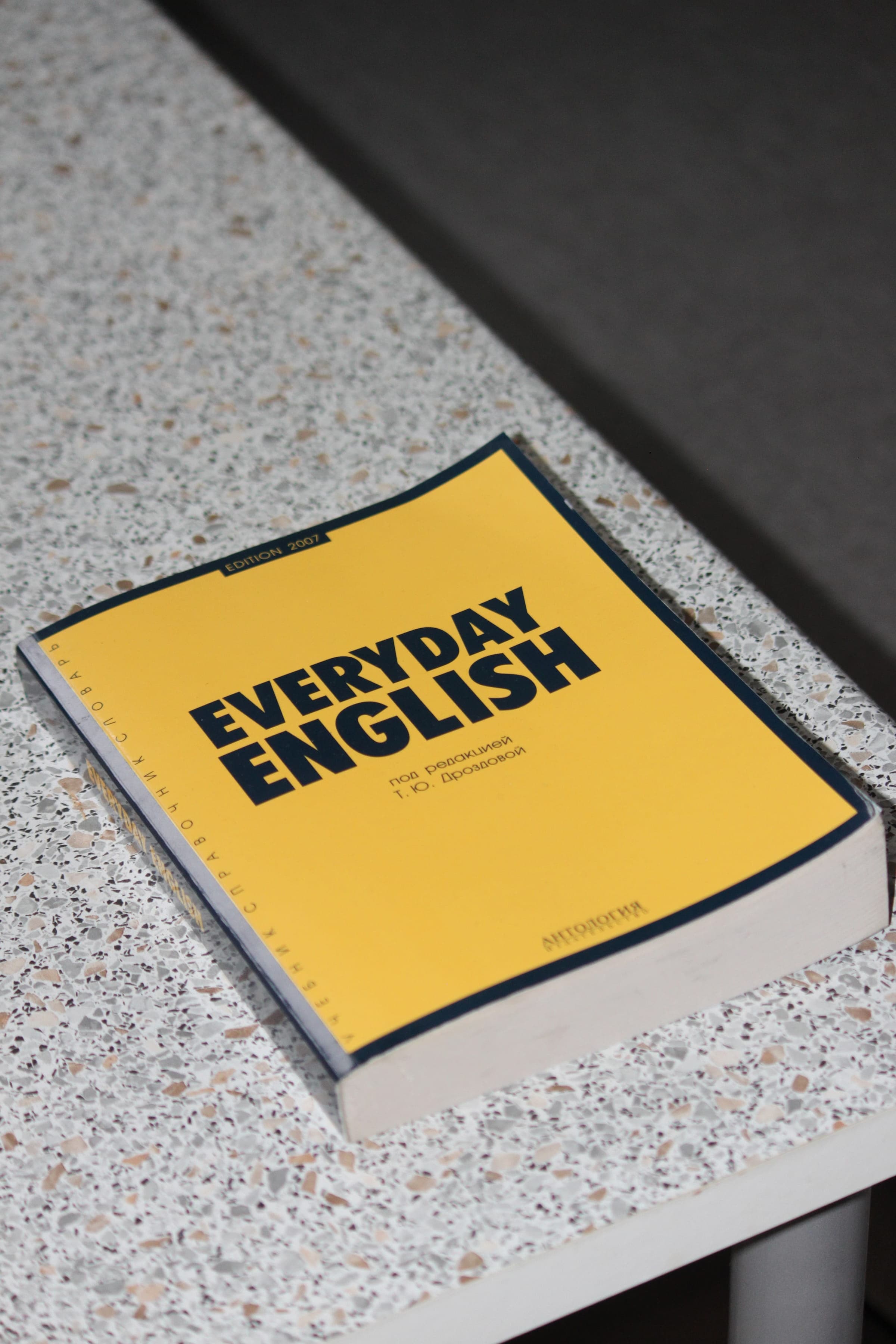
What's particularly fascinating about this moment in technological history is that we're not just deciding how to price a new product—we're essentially creating new economic models for a kind of value that's never existed before. The decisions we make now about how to price AI Agents will likely echo forward for decades, shaping not just how we use these technologies, but how we think about them, and ultimately, how we think about work itself.
And isn't that just the kind of thing that keeps you up at night, staring at the ceiling, wondering if somewhere out there an AI Agent is doing the same thing—minus the ceiling, of course.